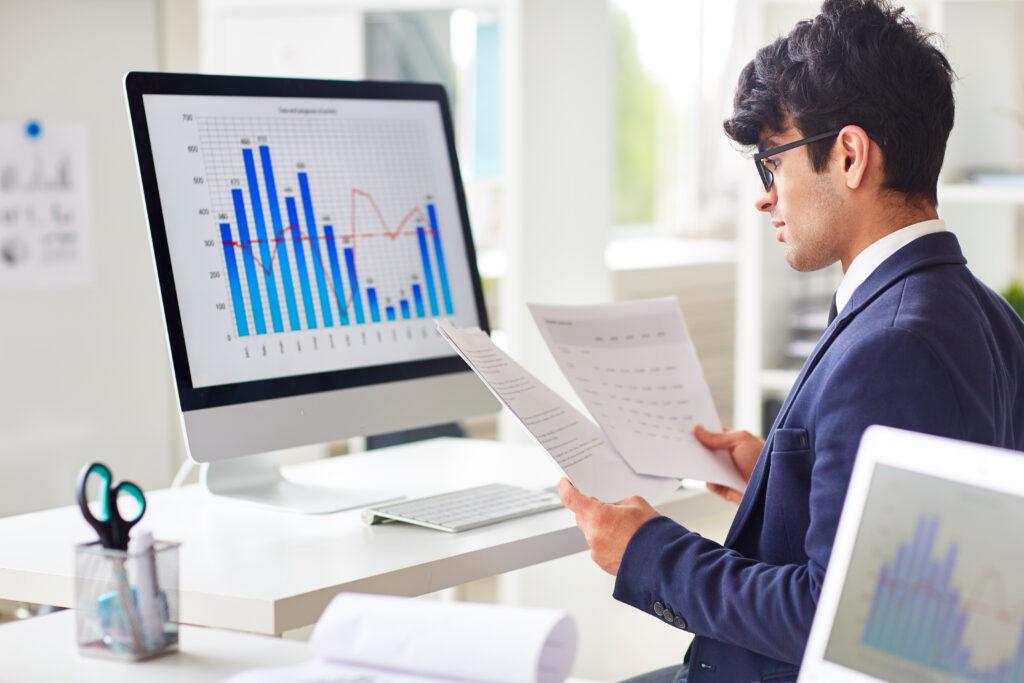
In the constantly advancing field of artificial intelligence (AI), quality and relevance of training data are vitally important to its successful development. Data annotation services play an essential part in this process by accurately labeling and annotating datasets to enable AI algorithms to learn effectively and make predictions effectively. In this comprehensive guide, we’ll delve into the importance and uses of data annotation services available, discuss their benefits and drawbacks, outline best practices for AI model training and demonstrate case studies of successful results, before concluding with strategies to maximize AI model performance through data annotation.
An Introduction to Data Annotation
Data annotation refers to the practice of labeling and categorizing data so it is accessible for artificial intelligence algorithms. Annotated data forms the backbone for AI model training – from learning patterns and predictions, through to accurately performing tasks – without proper annotation, AI models may produce unreliable results or fail to adapt to new situations; underscoring its criticality in AI development.
An Overview of Available Data Annotation Services Data annotation services encompass a diverse set of techniques and methodologies tailored to specific data types and AI applications. Some common types of annotation services offered today are:
Image Annotation: Labeling and outlining objects, shapes, and features within images for tasks such as object detection, image segmentation, and facial recognition.
Text Annotation: Tagging and classifying text data for tasks such as sentiment analysis, named entity recognition, and text classification.
Video Annotation: Annotating video data in real time in order to identify and track objects, actions, and events for applications like video surveillance and activity recognition.
Audio Annotation: Transcribing and annotating audio data to accomplish tasks such as speech recognition, speaker diarization and emotion detection.
Sensor Data Annotation: Labeling and annotating sensor data collected from IoT devices, wearables and other sources to support applications like predictive maintenance, anomaly detection and environmental monitoring.
Benefits of Utilizing Data Annotation Services
Data annotation services offer several advantages for AI model training, including:
Improved Model Accuracy: With accurate and relevant annotations, AI models learn from high-quality training data more efficiently, leading to more accurate predictions and higher performance.
Speedier Model Development: Outsourcing data annotation tasks to experienced service providers speeds up the model development process, enabling organizations to bring AI solutions faster to market.
Scalability: Data annotation services have the capacity to handle large volumes of data without needing additional infrastructure or resources, enabling organizations to annotate datasets of any size without investing in additional infrastructure or resources.
Cost-Effectiveness: Outsourcing data annotation tasks may be more cost-effective than hiring and training in-house annotators for short-term or specialized projects.
Challenges and Considerations in Data Annotation
While data annotation can provide numerous benefits, it also presents numerous obstacles and considerations that organizations must address to be successful with it. These issues include:
Quality Control: Accuracy and consistency across datasets is of critical importance in protecting the quality of training data, and avoiding bias or error in AI models.
Privacy and Security: Securing sensitive or personally identifiable data during annotation is vital to meeting data protection regulations and protecting against data breaches.
Annotation Variability: Different annotators may interpret and label data differently, creating variations and inconsistencies in annotations that can hinder model performance.
Annotation Tooling and Infrastructure: Selecting appropriate annotation tools and infrastructure is vital to creating efficient and effective data annotation, taking into account factors like usability, scalability and integration into existing workflows.
Best Practices for Effective AI Model Training
Organizations seeking to maximize the efficacy of their AI model training programs should adhere to these best practices:
Define Annotation Guidelines: Draft clear and comprehensive annotation guidelines in order to maintain consistency and accuracy across annotations, providing annotators with instructions and examples as references.
Quality Assurance: Establish effective quality assurance processes to validate annotations and detect discrepancies or errors, employing techniques such as inter-annotator agreement and consensus-based annotation.
Iterative Refinement: Evaluate and refine annotation guidelines on an ongoing basis in response to user feedback and performance metrics, improving their quality and relevance over time.
Collaborative Annotation: Foster collaboration and communication among annotators, data scientists, and domain experts to share knowledge, address challenges, and establish standard annotation procedures and best practices.
Training and Feedback: Engage annotators in regular training and feedback programs designed to build their skillset and ensure they remain proficient and consistent with their annotation tasks.
Case Studies Showcase Successful Outcomes
Multiple case studies showcase the transformative impact of data annotation services on AI model training and deployment:
Medical Image Analysis: A healthcare organization collaborated with a data annotation service provider to annotate a large dataset of medical images to train an AI model to detect cancerous lesions more accurately, leading to more accurate diagnoses and improved patient outcomes. With an accurate annotated dataset in hand, their AI model could achieve high sensitivity and specificity rates when detecting tumors resulting in more precise diagnoses and better patient outcomes.
Autonomous Vehicles: A leading automotive company collaborated with a data annotation service provider to annotate millions of images and videos for training autonomous driving systems. The annotated dataset enabled AI algorithms capable of recognizing traffic scenarios more reliably – further increasing safety and reliability with self-driving vehicles.
Natural Language Processing (NLP): A technology startup collaborated with a data annotation service provider to annotate a large corpus of text data for use in training a natural language processing (NLP) model. Using this annotated dataset, the NLP model could accurately extract information from unstructured text documents thereby improving document processing and information retrieval tasks.
These case studies demonstrate how data annotation services can create positive results across a range of AI applications, from healthcare and automotive to natural language processing and beyond. By employing experienced annotation services, organizations can successfully address challenges, accelerate model development and deliver superior performance in their AI initiatives.
Conclusion: Maximizing AI Model Performance With Annotation
Data annotation services play a vital role in streamlining AI model training and assuring its accuracy and reliability. By accurately labeling and annotating datasets, organizations can give AI systems access to high-quality training data that they require for learning and generalizing effectively. Despite any challenges or considerations associated with data annotation services, their benefits-such as improved model accuracy, faster development cycles, scalability, cost-effectiveness–far outweigh their downsides.
Organizations looking to optimize AI models should follow best practices such as setting annotation guidelines, implementing quality assurance processes, encouraging collaboration among annotators and providing regular training and feedback sessions. Incorporating case studies and success stories as evidence can instil confidence in data annotation services while showing their transformative effects across industries.
Data annotation services are invaluable tools for organizations seeking to implement AI solutions that provide accurate predictions, actionable insights, and tangible value. By adopting data annotation as a means of improving AI model performance, organizations can tap the full potential of artificial intelligence while driving innovation and competitiveness in today’s digital environment.