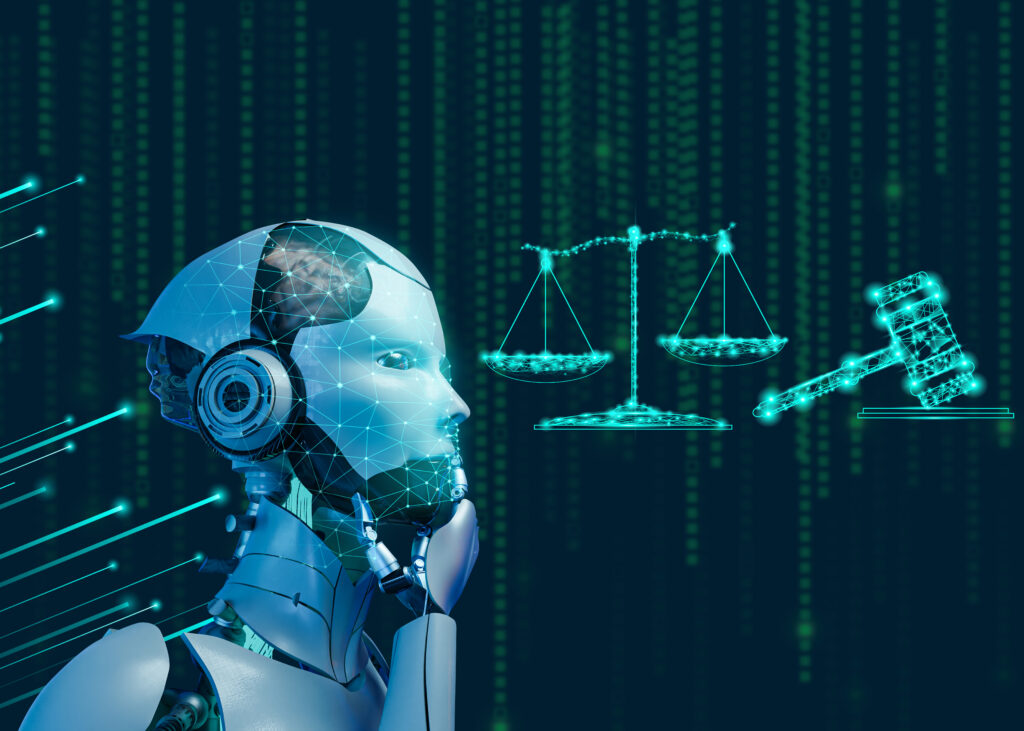
As businesses seek ways to use data more effectively for competitive advantage, machine learning (ML) has emerged as a useful strategy. By mining data to extract actionable insights and inform strategic decision-making processes, Machine Learning allows organizations to optimize operations, enhance customer experiences, and drive innovation. In this comprehensive guide, we’ll explore the role and benefits of machine learning in business operations, from its benefits to understanding data to implementation techniques and tools for machine learning (ML). In particular, we will delve into understanding data usage processes, discuss techniques for using it for data interpretation and storage purposes as well as ways ML can provide competitive advantage; address challenges to implementation processes while showing real-life examples of its success; finally highlighting overarching benefits derived from using it effectively in business operations.
Machine Learning Is Essential to Business Machine learning (ML) has become an essential component of success in today’s digital economy, due to the exponential expansion of data and complexity in modern environments. Leveraging its power, machine learning can extract actionable insights, predict future trends, optimize decision-making processes and unlock their full potential to gain competitive edge within industries.
Understanding Data and Leveraging It Effectively
An essential element of successful machine learning initiatives is understanding and using data effectively. Organizations must take an analytical approach when collecting, cleaning and analyzing customer behavior data from various sources in order to gain actionable insights about customer behavior, market trends and operational performance. By using techniques such as data mining, exploratory data analysis and predictive modeling techniques they can uncover hidden patterns, correlations or opportunities within their data that lead to informed decision-making and strategy development.
Implementing Machine Learning: Tools and Techniques
Implementing machine learning within a business setting requires selecting appropriate tools and techniques tailored to specific use cases and objectives. Common machine learning (ML) techniques and tools include:
Supervised Learning: Training machine learning models to make predictions or classifications on labeled data to make predictions or classifications based on input features and target variables.
Unsupervised Learning: Exploring patterns and structures within data without explicit supervision, such as clustering similar data points together or decreasing dimensionality.
Deep Learning: Deep learning utilizes neural networks with multiple layers to learn complex representations of data and extract high-level features for tasks such as image recognition, natural language processing and recommendation systems.
Tools: Utilizing popular Machine Learning frameworks and libraries such as TensorFlow, PyTorch, Scikit-learn and Keras to quickly develop and deploy machine learning models efficiently and effectively.
Machine Learning Enhances Decision-Making and Strategy
Machine learning gives businesses a significant competitive edge by offering more accurate predictions, faster insights, and smarter decision-making. By processing vast amounts of data in real-time, machine learning algorithms can identify market trends, customer preferences, and emerging opportunities – helping organizations adapt quickly and stay ahead of competition. Furthermore, machine learning-driven optimization techniques can increase operational efficiencies while simultaneously decreasing costs while improving overall customer experiences to further bolster a company’s position within its market.
Implementation Challenges and Overcome Obstacles
Although machine learning offers immense potential benefits to organizations, its implementation poses several obstacles that they must surmount in order to be successful – these include:
Data Quality: Ensuring data’s quality, accuracy, and completeness is of utmost importance in order to achieve successful ML initiatives; poor-quality data may lead to biased models, inaccurate predictions, or suboptimal outcomes that compromise success
Skills Gap: Employing and sustaining a workforce capable of designing, implementing and interpreting machine learning (ML) solutions requires specialists with skills in mathematics, statistics, programming languages, domain knowledge and domain expertise – these may not exist in all organizations.
Resource Constraints: Investing in infrastructure, technology and human capital needed for Machine Learning initiatives can be costly and time consuming for smaller businesses with limited budgets and capabilities.
Ethical Considerations: Considering ethics and regulatory concerns related to data privacy, security, and fairness when developing machine learning models is vitally important in order to ensure they are created responsibly and ethically.
Case Studies of Machine Learning Success
Numerous real-world case studies illustrate the profound effect that machine learning can have on business operations and results. Some notable examples are:
Netflix uses Machine Learning algorithms to analyze viewer preferences and behavior data in order to personalize content recommendations and increase user engagement.
Amazon uses machine learning (ML)-powered recommendation systems to offer personalized suggestions based on customer browsing and purchasing behavior, leading to increased sales and greater customer satisfaction.
Google: Utilizing machine learning (ML) techniques to strengthen search engine algorithms, deliver relevant search results and enhance voice recognition and natural language processing capabilities.
Uber uses machine learning models to optimize ride-hailing routes, predict demand patterns, and dynamically adapt pricing algorithms – leading to more efficient operations and enhanced customer experiences.
Conclusion: Benefits of Leveraging Machine Learning in Business
Machine learning offers businesses many advantages, from enhanced decision-making and strategy development, to improved operational efficiencies and customer satisfaction. Organizations can harness data effectively and implement machine learning (ML) techniques and tools to unlock valuable insights, uncover new opportunities, and drive innovation within their industries. Machine learning holds immense promise for business operations despite any obstacles it may present, helping organizations thrive in today’s increasingly data-driven and competitive marketplace. By investing in machine learning capabilities and expertise, organizations will open up endless possibilities for using data strategically for strategic advantage and sustainable growth.