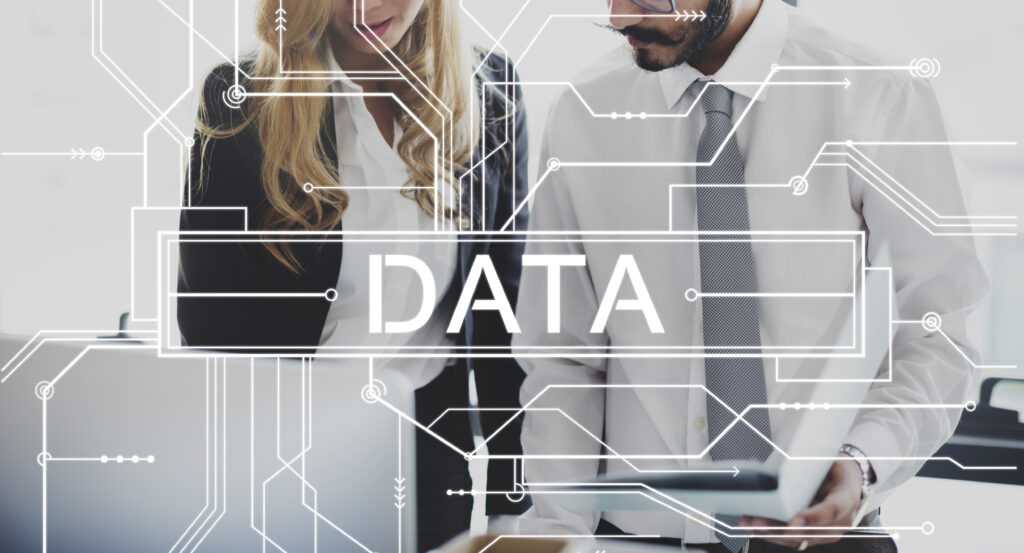
In the realm of machine learning and artificial intelligence, data is the lifeblood. However, raw data alone is often insufficient for training algorithms. This is where data labeling steps in. In this comprehensive guide, we delve into the intricacies of data labeling, its importance, and the best practices to ensure accurate annotations.
Understanding Data Labeling
Data labeling, also known as data annotation, involves assigning meaningful tags or labels to raw data, making it understandable for machines. Whether it’s images, text, audio, or video data, labeling provides context and clarity, enabling machine learning algorithms to learn and make informed decisions.
Importance of Accurate Data Labeling
Accurate data labeling lays the foundation for successful machine learning models and AI systems. Without precise annotations, models may produce erroneous results, leading to flawed decisions. It’s crucial for training algorithms effectively and ensuring reliable performance.
Best Practices for Data Labeling
Define Clear Guidelines: Establish comprehensive guidelines for labeling data to maintain consistency and accuracy.
Implement Quality Control: Incorporate robust quality control mechanisms to validate labeled data and detect any discrepancies.
Iterative Process: Treat data labeling as an iterative process, continuously refining and updating labels to enhance model performance.
Utilize Domain Experts: Engage domain experts to ensure accurate labeling and interpretation of data, particularly in specialized fields.
Leverage Data Augmentation: Employ data augmentation techniques to expand the labeled dataset, improving model robustness and generalization.
Tools and Technologies for Data Labeling
Efficient data labeling relies on advanced tools and technologies:
Labeling Platforms: Platforms like Labelbox, Scale AI, and Supervised offer intuitive interfaces and collaboration features for efficient annotation.
Automated Labeling: AI-powered tools such as Snorkel and LabelIng automate labeling processes, speeding up annotation tasks.
Crowdsourcing Platforms: Services like Amazon Mechanical Turk and CrowdFlower provide access to a global pool of annotators for large-scale labeling projects.
Challenges in Data Labeling
Despite its importance, data labeling poses several challenges:
Scalability: Scaling labeling efforts to handle large datasets efficiently can be challenging.
Annotation Errors: Human errors in labeling can impact the quality and reliability of training data.
Cost Considerations: Labeling can be resource-intensive, with costs associated with tools, labor, and quality control measures.
Harnessing the Power of Data
In conclusion, data labeling is a critical step in unlocking the potential of machine learning and artificial intelligence. By understanding its importance and implementing best practices and tools, organizations can harness the power of data to drive innovation, make informed decisions, and stay ahead in today’s competitive landscape.